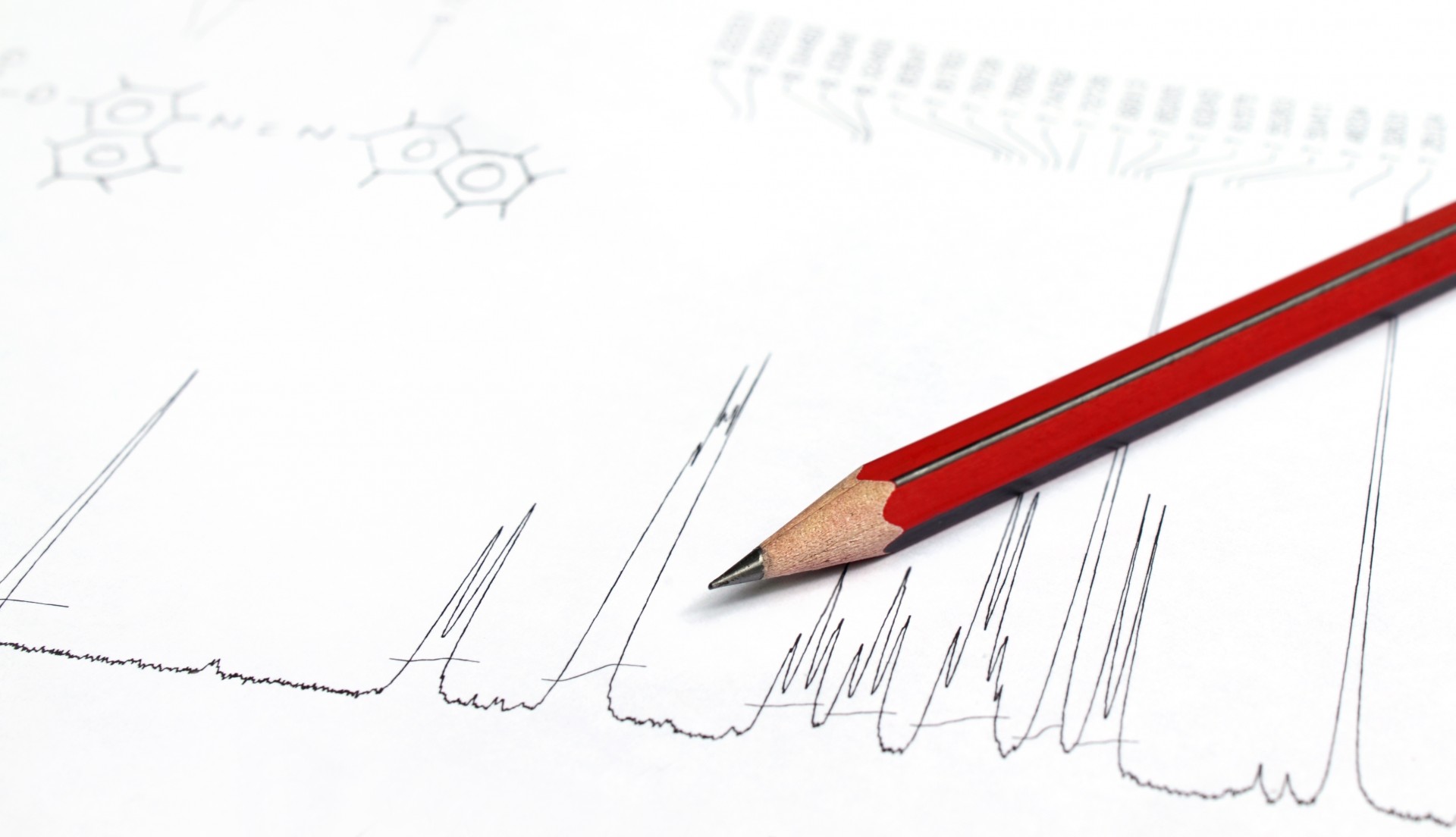
Using this method, data from NMR spectrometers may be analysed faster and with greater accuracy.
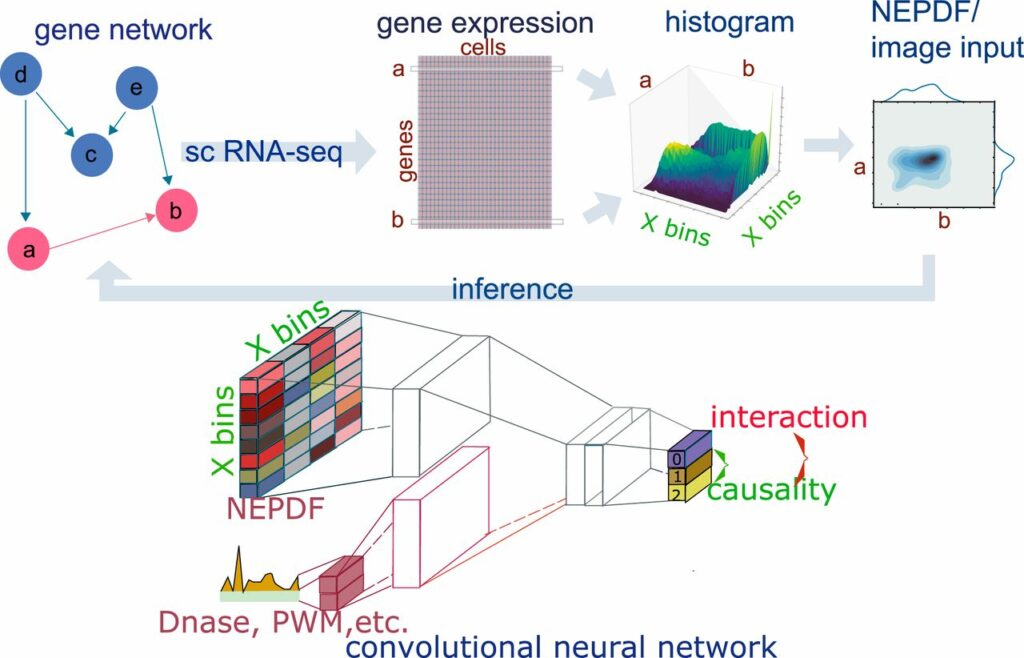
Scientists have devised a method for better analysing data from a strong scientific tool: nuclear magnetic resonance. The method is based on machine learning (NMR). In order to better understand proteins and chemical interactions in the human body, NMR data can be used in a variety of ways. NMR is a medical diagnostic technique that is closely related to magnetic resonance imaging (MRI).
Scientists may determine the structure of molecules such as proteins using NMR spectrometers, however it can take a significant amount of time for highly skilled human professionals to examine the data generated by these instruments. This new machine learning technology can examine the data considerably more rapidly and just as correctly.
Researchers explained their approach in a report that was just published in Nature Communications. The process, in essence, teaches computers to disentangle complex data regarding atomic-scale features of proteins, parsing it into individual, readable images.
“In order to be able to use these data, we must first separate them into features from different parts of the molecule and quantify their specific properties,” said Rafael Brüschweiler, senior author of the study, Ohio Research Scholar, and professor of chemistry and biochemistry at The Ohio State University. “We must first separate them into features from different parts of the molecule and quantify their specific properties.” It was previously extremely difficult to utilise computers to distinguish between these particular traits when they overlapped, according to the researcher.
The approach, which was devised by Dawei Li, the study’s lead author and a research scientist at Ohio State’s Campus Chemical Instrument Center, teaches computers how to scan images from NMR spectrometers, according to the researchers. This type of image, known as a spectrum, appears as hundreds and thousands of peaks and troughs, and it can be used to illustrate changes in proteins or complex metabolite mixes in a biological sample, such as blood or urine, at the atomic level, among other things. The NMR data provide significant information about the function of a protein as well as important signals about what is going on in a person’s body, according to the researchers.
Deconstructing the spectra into legible peaks, on the other hand, might be problematic because the peaks frequently overlap. A mountain range-like impression is created, with lesser peaks that may also contain vital information being obscured by larger peaks that are closer to the viewer.
According to Brüschweiler, NMR spectra are analogous to the QR code readers on your phone: “NMR spectra are like a QR code for molecules— every protein has its own distinctive ‘QR code,'” he explained. “It should be noted, however, that the individual pixels of these ‘QR codes’ might significantly overlap with one another. There’s no way your phone would be able to understand them. And that is the challenge we have had with NMR spectroscopy and that we were able to fix by teaching a computer to accurately read these spectra.”
The procedure entails the creation of an artificial deep neural network, which is a multi-layered network of nodes that the computer utilises to separate and analyse large amounts of information.
This network was developed by the researchers, who then trained it how to evaluate NMR spectra by feeding spectra into the computer that had already been studied by a person and told the computer that the previously known right result was to be used. The procedure of training a computer to evaluate spectra is similar to that of teaching a child to read — the researchers started with extremely simple spectra and worked their way up to more complex ones. As soon as the computer grasped this concept, the researchers went on to more complex sets of data. In the end, they supplied the computer very complicated spectra of several proteins as well as a mouse urine sample to see what happened.
As a result of utilising a deep neural network that had been trained to assess spectra, the researchers discovered that their computer was capable of parsing out the peaks in the extremely complicated sample with the same accuracy as a human expert. Furthermore, the machine completed the task more quickly and with greater consistency.
According to Brüschweiler, using machine learning as a tool to evaluate NMR spectra is only one essential step in the lengthy scientific process of NMR data interpretation, which can take years. However, this research boosts the capabilities of NMR spectroscopists, especially those who will use Ohio State’s new National Gateway Ultrahigh Field NMR Center, which was supported by the National Science Foundation with a $17.5 million grant last year. The centre, which is set to open its doors in2022, will be home to the first 1.2 gigahertz NMR spectrometer in North America, among other firsts.
The National Science Foundation and the National Institutes of Health provided financial support for this research.
Others at Ohio State’s Campus Chemical Instrument Center who were involved in this study include Alexandar Hansen, Chunhua Yuan, and Lei Bruschweiler-Li, all of whom are also research scientists.
For More Information Visit : Theny Magazine